Understanding SEO: Using Secondary Dimensions for Boosted Analytics
Understanding SEO: Using Secondary Dimensions for Boosted Analytics
Blog Article
Discover Deeper Insights With Additional Dimensions
Secondary dimensions offer as an effective device in the realm of data evaluation, providing a nuanced perspective that goes beyond surface-level observations. Remain tuned to uncover how secondary measurements can transform the method you analyze and take advantage of your information for strategic advantages.
Advantages of Additional Measurements
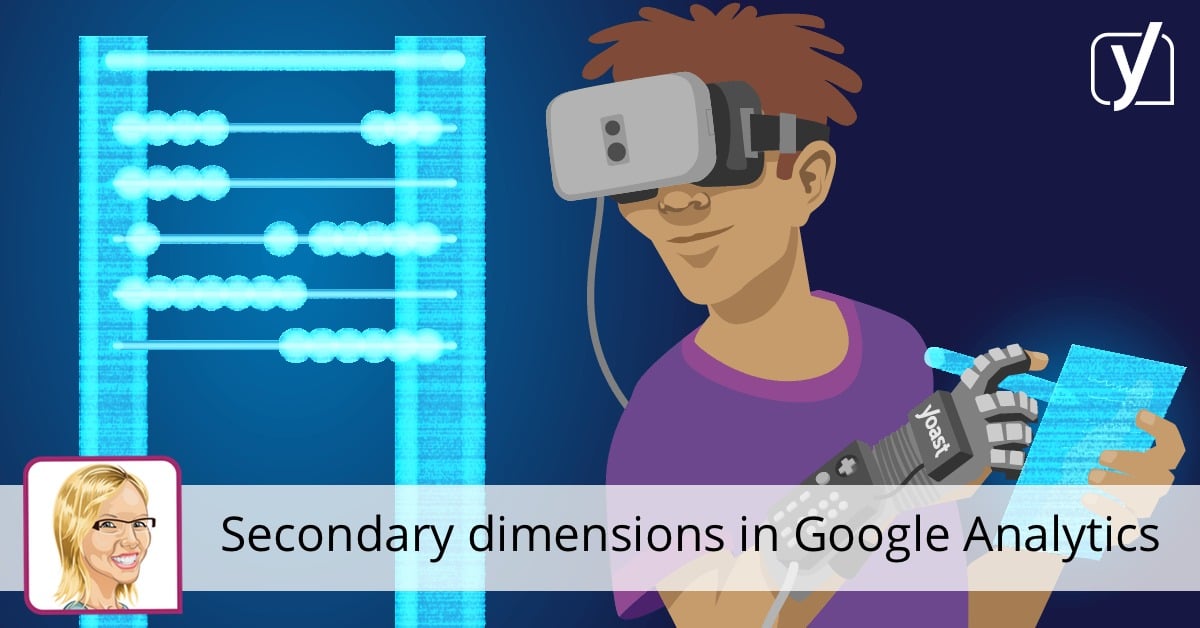
One of the key benefits of secondary measurements is the capability to enhance the context of the primary data. This added context enables experts to attract even more accurate verdicts and make notified choices based on a more thorough view of the data. Additional dimensions help in supplying a more holistic view of the relationships between different variables, consequently aiding in the identification of underlying aspects that might influence the main dataset.
Basically, additional measurements play a vital role in enriching data evaluation processes, providing a much more nuanced point of view that can cause workable referrals and valuable insights.
Application Tips for Second Measurements
Implementing secondary dimensions effectively requires a tactical strategy that straightens with the specific objectives of the data analysis procedure. To begin, it is crucial to clearly specify the goals of the analysis and determine the vital metrics that will certainly provide the most important insights - secondary dimensions. Select secondary measurements that match the main dimensions and aid in revealing much deeper patterns or connections within the information
When applying additional dimensions, it is important to consider the context in which the evaluation will be conducted. Recognizing the target market and their information demands will certainly direct the selection of pertinent additional measurements that include meaningful context to the key information points. In addition, guarantee that the secondary measurements chosen are suitable with the main dimensions and can be effectively contrasted or incorporated to extract valuable understandings.
Moreover, it is advised to evaluate different combinations of primary and secondary dimensions to discover numerous perspectives and uncover surprise connections within the data. Frequently examining and improving the choice of second dimensions based upon the developing logical requirements will ensure the evaluation remains insightful and appropriate.
Studying Information With Secondary Dimensions
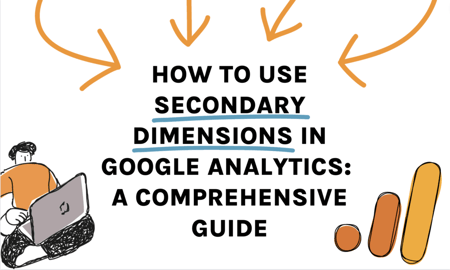
When examining data with second measurements, it is vital to think about how various variables engage with one another. By cross-referencing key data with second measurements, experts can discover correlations and reliances that supply an even more all natural view of the data. This strategy not only boosts the accuracy of understandings but additionally helps in making even more informed decisions based upon the findings.
Moreover, examining information with second measurements makes it possible for the recognition of outliers or abnormalities that might influence the total evaluation. By diving deeper right into the data with additional dimensions, analysts can gain a more profound understanding of the hidden variables driving the fads observed in the key dataset.
Making The Most Of Insights With Secondary Dimensions
To extract a greater degree of deepness and accuracy from data analysis, leveraging secondary measurements is extremely important for making best use of understandings. By including second measurements into your analysis, you can reveal beneficial partnerships and patterns that may not be quickly noticeable when checking out data through a key measurement alone. Secondary measurements enable you to slice and dice your information additionally, offering a more thorough understanding of the aspects influencing your metrics.
When made use of successfully, additional dimensions can enhance the context of your main data, supplying a much more nuanced perspective on your evaluation. By adding additional dimensions such as location, customer, or time demographics, you can get a deeper understanding of just how different sections communicate with your web content or products.
In addition, additional measurements can help you determine outliers, trends, and relationships that could or else go undetected. By exploring your information from multiple angles, you can remove richer insights and make even more enlightened choices based upon a comprehensive understanding of the underlying factors at play.
## Common Errors to Prevent When Using Secondary Measurements
When incorporating additional dimensions right into information evaluation, it is vital to be conscious of usual blunders that can prevent the removal of valuable insights. One prevalent error is the misuse of secondary dimensions without a clear goal in mind. It is vital to specify details objectives and inquiries prior to picking second measurements to guarantee they straighten with the evaluation objective.
An additional blunder to stay clear of is overcomplicating the evaluation by consisting anchor of also several secondary measurements at visit their website the same time. This can cause info overload and make it testing to draw significant final thoughts from the data. It is a good idea to begin with a couple of appropriate additional dimensions and slowly integrate much more as needed.
Additionally, neglecting data integrity issues can substantially influence the precision of insights derived from second measurements. Inaccurate or insufficient information can distort the analysis results and mislead decision-making processes. Frequently validating and cleansing the data is crucial to make certain the integrity of the insights generated.
Conclusion
To conclude, the calculated usage of second measurements in data evaluation provides an effective tool for unlocking much deeper insights and boosting decision-making procedures. By including added layers of information, experts can obtain an extra extensive understanding of their dataset, reveal hidden patterns, and identify essential factors influencing end results. Through cautious consideration and execution of additional measurements, researchers can make the most of the worth of their data and drive notified decision-making in numerous fields.
Select additional measurements that complement the main measurements and help in discovering much deeper patterns or connections within the information.
Additionally, make sure that the second dimensions selected are suitable with the primary dimensions and can be properly compared or incorporated to remove important understandings.
Utilizing second that site dimensions in information evaluation enhances the depth and breadth of understandings derived from the main information points. By cross-referencing primary data with second measurements, analysts can reveal correlations and dependencies that provide an even more holistic view of the data. By including secondary measurements right into your evaluation, you can reveal important connections and patterns that might not be immediately apparent when looking at data with a key measurement alone.
Report this page